
【Domestic Papers】Jinling Institute of Technology --- Increased thermal conductivity of β-Ga₂O₃ using Al substitution: Full spectrum phonon engineering
日期:2025-03-24阅读:257
Researchers from the Jinling Institute of Technology have published a dissertation titled "Increased thermal conductivity of β-Ga2O3 using Al substitution: Full spectrum phonon engineering" in Journal of Applied Physics.
Acknowledgments
This work was financially supported by the Science Foundation of Jinling Institute of Technology (No. jit-rcyj-202001) and the scholarship from China Scholarship Council (CSC) under Grant No. 202308320197. Professor Chang is working at the Nanyang Technological University supported by the National Research Foundation, Singapore, under its Fellowship Award (No. NRF-NRFF13-2021-0010), the Agency for Science, Technology and Research (A*STAR) under its Manufacturing, Trade and Connectivity (MTC) Individual Research Grant (IRG) (Grant No. M23M6c0100), the Singapore Ministry of Education (MOE) Academic Research Fund Tier 3 Grant (No. MOE-MOET32023-0003), the Singapore Ministry of Education (MOE) AcRF Tier 2 Grant (No. MOE-T2EP50222-0014), and the Nanyang Assistant Professorship Grant (NTU-SUG).
Background
Gallium oxide (β-Ga2O3) is drawing widespread attention in the field of power electronics primarily due to its ultra-wide bandgap of approximately 4.8–4.9 eV, which is significantly higher than that of GaN (3.4 eV). The ultra-wide bandgap enables β-Ga2O3 devices to operate at an exceptionally high critical electric field (∼8 MV/cm), far exceeding GaN (∼3.3 MV/cm). This allows for the fabrication of devices that can sustain higher voltages with smaller geometries, enhancing energy efficiency and reducing size. Besides, β-Ga2O3 can be easily deposited using magnetron sputtering in a low vacuum condition without worrying about oxygen, simplifying the process.
Abstract
Improving the thermal conductivity of β-Ga2O3 is critical for optimizing its performance in high-power electronic devices, as effective thermal management significantly influences their output power and reliability. In this work, the thermal conductivities of β-Ga2O3 and (AlxGa1−x)2O3 alloys along the (-201) direction were first computed using a non-equilibrium molecular dynamics method based on the deep learning potential. Our results indicate that the calculated thermal conductivity of β-Ga2O3 is 16.6 W m−1K−1 along the (-201) direction, which is in excellent agreement with experimental measurements. In our findings, an Al to Ga ratio of 1:1 leads to the thermal conductivity of the (AlxGa1−x)2O3 alloy being more than twice that of β-Ga2O3, regardless of the Al substitution sites. The (Al0.5Ga0.5)2O3 alloy exhibits enhanced thermal conductivity due to the improved transport properties of optical phonon modes, including the increased group velocities, the enhanced participation, and the induced new vibrational modes at higher frequencies. This research provides theoretical predictions regarding the optimal Al to Ga ratio to enhance the thermal conductivity of (AlxGa1−x)2O3 alloys, offering crucial insights for the design and thermal management of β-Ga2O3 power devices.
Conclusion
In conclusion, we conducted numerical training based on deep neural network models to accurately describe the interactions between atoms in β-Ga2O3 and (AlxGa1−x)2O3 alloys. By establishing potential functions that characterize the energy and forces acting on the atoms, we were able to perform the NEMD simulations of thermal conductivities for both β-Ga2O3 and (AlxGa1−x)2O3 alloys along the (-201) direction. Our calculated thermal conductivity for β-Ga2O3 is 16.6 W m−1K−1, which aligns well with experimental values.
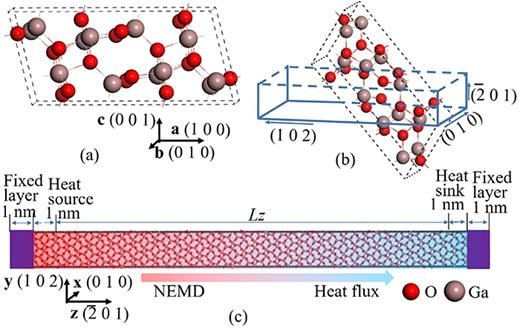
FIG. 1. (a) Conventional unit cell of β-Ga2O3, (b) the cleaved (-201) plane of β-Ga2O3, and (c) the model for computing the thermal conductivity along the (-201) direction based on the NEMD method.
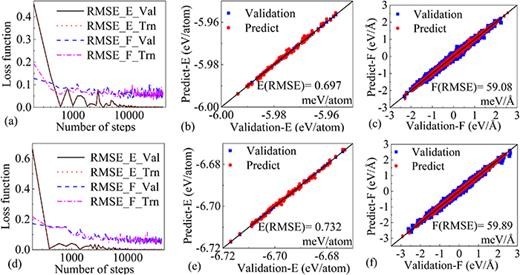
FIG. 2. (a) Loss function of the root-mean-squared error (RMSE) of energy (E) and force (F) in the training and validation datasets for β-Ga2O3. Comparison between prediction datasets using the deep learning potential and validation datasets from the AIMD calculation of (b) energy/atom and (c) force on atom for β-Ga2O3. (d) Loss function of the RMSE of energy and force in the training and validation datasets for (Al0.5Ga0.5)2O3 alloy. Comparison between prediction datasets using the deep learning potential and validation datasets from the AIMD calculation of (e) energy/atom and (f) force on atom for (Al0.5Ga0.5)2O3 alloy.
DOI:
doi.org/10.1063/5.0249882