
【International Papers】Optimizing the morphology transition on MOVPE-grown (100) β-Ga₂O₃ film between step-flow growth and step-bunching: A machine learning-assisted approach
日期:2025-05-15阅读:25
Researchers from the Leibniz-Institut für Kristallzüchtung (IKZ) have published a dissertation titled "Optimizing the morphology transition on MOVPE-grown (100) β-Ga2O3 film between step-flow growth and step-bunching: A machine learning-assisted approach" in APL Materials.
Background
Recently, β-Ga2O3 has attracted great attention in both academia and industry as one of the potential candidates for power electronics applications due to its wide bandgap of 4.9 eV and a high theoretical breakdown field of up to 8 MV/cm. A critical advantage of β-Ga2O3 is the availability of large, high-quality native substrates grown from the melt using various techniques, such as the Czochralski method, edge-defined film-fed growth (EFG), and the vertical Bridgman method, which allows for the economically practical manufacturing of β-Ga2O3 compared to other wide-bandgap semiconductors. Due to these excellent physical properties, β-Ga2O3 is considered as a possible replacement of GaN and SiC for future power electronics.
To fully realize the potential of β-Ga2O3 in power electronics, it is crucial to produce high-quality epitaxial layers. The most investigated substrate orientations are (100), (010), and (001). Among these, the (100) plane is the preferred cleavage plane of β-Ga2O3 due to its lowest surface energy and ease of preparation. However, achieving high-quality (100) β-Ga2O3 films remains a significant challenge. This difficulty arises from the high density of twins and stacking faults in the grown layers caused by the double positioning of Ga adatoms on the (100) plane. These structural defects complicate characterization during the epitaxy process. Addressing this issue requires identifying and understanding the key factors that influence the growth process under varying conditions, especially those that impact surface morphology—a critical determinant of the electrical performance and overall quality of the fabricated devices. Among all available growth techniques, metalorganic vapor phase epitaxy (MOVPE) stands out due to its advantages for large-scale device fabrication, including large epitaxy areas, good reproducibility, and high deposition rates.
Abstract
The step-bunching instability in (100) β-Ga2O3 films grown via metalorganic vapor phase epitaxy was investigated using a machine learning approach based on Random Forest (RF). This study reveals the interplay of Ga supersaturation (O2/Ga) and impurity effects as coexisting mechanisms driving the morphological transition (from step-flow growth to step-bunching). The developed machine-learning framework accurately classifies growth morphology and offers valuable insights by correlating theoretical principles with experimental parameters. Critical growth parameters influencing the film morphology were identified. The corresponding strategy, high Ga supersaturation, is proposed to mitigate the step-bunching formation and maintain the desired step-flow growth mode. Despite the challenges posed by small datasets, the RF model demonstrates robust classification performance, establishing machine learning as a powerful tool for experimental science.
CONCLUSION
In this study, the classical machine learning algorithm, Random Forest (RF), is applied to an experimental dataset of MOVPE-grown (100) β-Ga2O3 films on a vicinal substrate to investigate the mechanisms behind step-bunching formation. The RF model explores the complex interactions between growth parameters, such as Ga supersaturation (O2/Ga flux ratio) and impurity concentration (doping), to identify their roles in the transition from step-flow to step-bunching growth modes. By leveraging the RF algorithm, we pinpoint critical growth conditions that influence morphological stability, offering predictive insights into the process. These findings enable the development of strategies—such as maintaining high Ga supersaturation—to mitigate step-bunching by promoting the formation of a potential Ga adlayer, thus overcoming the step-pinning induced by the dopants (impurities) and the Ehrlich–Schwoebel barrier. This study highlights the power of machine learning as a robust tool for decoding complex growth dynamics in epitaxial film fabrication, even when working with limited datasets.
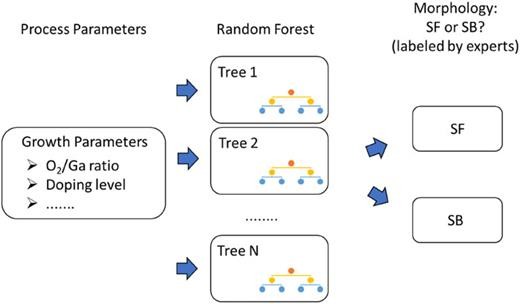
FIG. 1. Protocol to classify the film morphology with a trained RF model used in this work. The morphology was categorically labeled by epitaxy experts using a consistent annotation approach.
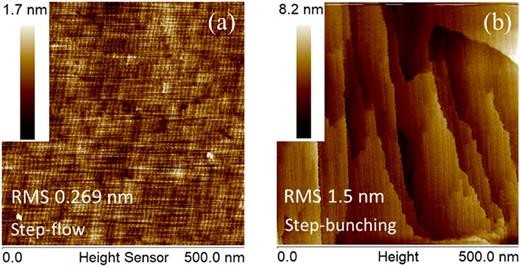
FIG. 2. Surface morphologies of (100) β-Ga2O3 films on (100) β-Ga2O3 4° off substrates observed by AFM: (a) step-flow and (b) step-bunching.
DOI:
doi.org/10.1063/5.0261944